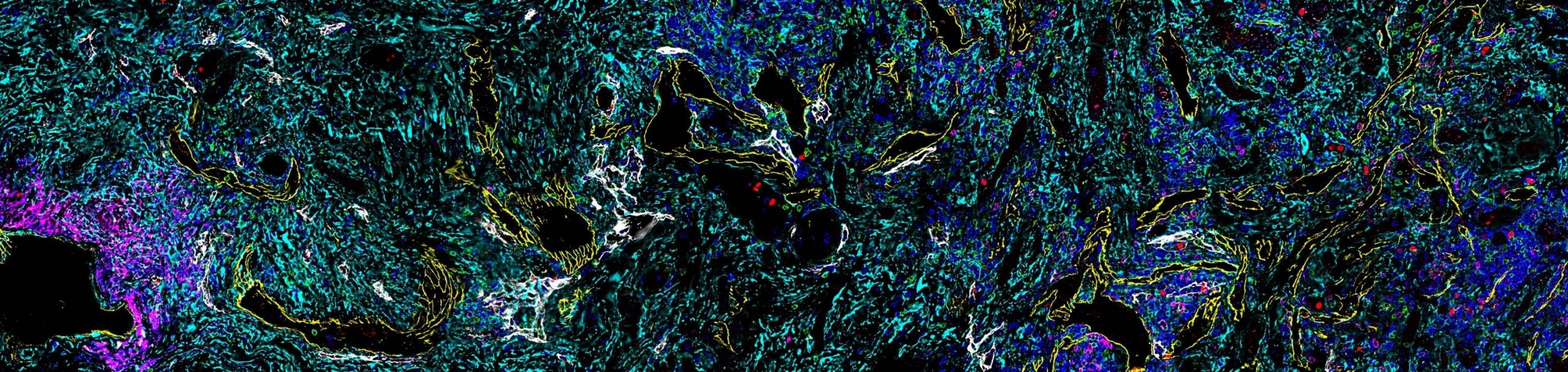
Spatial biology is adding new dimensions to translational medicine.
Spatial biology is adding new dimensions to translational medicine.
Advances in biomarker analysis have led to major breakthroughs in cancer research, translational medicine, therapy and diagnostics. Progress has been powered by rapid data generation using high throughput methods such as next generation sequencing and proteomics based on mass spectrometry. With much of the low hanging fruit already harvested, innovative therapies, such as immunotherapy, are offering new ways to combat cancer at the level of the individual patient. Biomarkers help to fine tune this personalized, or precision, medicine in the form of companion diagnostics that guide the use of personalized treatment options, for example in breast cancer.
Progress now depends on going to the next level – mapping biomarkers in space and time to elucidate the evolving tumor microenvironment (TME). This is where spatial biology and spatial-omics (or spatial multiomics), which integrates and visualizes transcriptomic and proteomic data, are providing invaluable insights.
Spatial-omics – mapping the progress of cancer in 3D
The diverse individual cell types and cell states of tumors create a highly complex and dynamic TME that can only be fully understood by spatial analysis. Spatial-omics is now extending the resolution of cancer research and diagnosis from the tissue or organ to the cellular and subcellular level. Spatial phenotyping, based on techniques such as multiplex immunohistochemistry (IHC) or multiplex immunofluorescence (mIF), is proving to be particularly valuable and involves tissue imaging with single cell resolution to visualize and quantitate biomarker expression to map the organization of cells in the tissue and how they interact.
Combining spatial-omics data, such as gene expression data and protein co-detection data can add a new dimension of information – for example, patterns and associations formed by individual cells with specific phenotypes and functions that are not evident with other methodologies (cellular neighborhoods), and posttranslational modification and subcellular localization of proteins and their dysregulation in disease. Spatial transcriptomics and spatial phenotyping are also supporting the drive toward personalized (individualized) medicine.
Spatial phenotyping brings new insights and increases predictive power
There are many examples of how combining biomarkers can bring new insights into disease and therapeutic mechanisms and increase predictive power. Spatial phenotyping, for example based on multiplex IHC, promises to bring this to a new level. For example, cancer immunotherapy is revolutionizing cancer treatment by boosting the natural immune response and advances depend on a deep understanding of the TME. Multiplexed immunophenotyping can give new insights into this and are essential in the identification of predictive biomarkers of response and gaining insights into therapeutic mechanisms of action1.
One example that illustrates the predictive power of spatial phenotyping is the meta-analysis by Steve Lu and coworkers, based at Johns Hopkins Medical Institutions, Baltimore, USA aimed at determining the value of this approach in predicting patient response to anti-PD-1/PD-L1 (programmed cell death ligand 1) therapy2. Their analysis built on reports on the diagnostic accuracy of tumor mutational burden (TMB), gene expression profiling (GEP), and spatial phenotyping using multiplex immunohistochemistry/immunofluorescence (mIHC/IF). The meta-analysis showed that while TMB, PD-L1 IHC, and GEP alone had comparable areas under the curve (AUC) in predicting response to treatment, mIHC/IF and multimodality biomarker strategies were better at predicting response (Figure 1).
Figure 1. Receiver operating characteristic (sROC) curves used to compare performance of the different biomarker approaches in predicting response to treatment. The relative area under the curves (AUCs) measure each approach’s ability to distinguish responders from non-responders. Spatial phenotyping using multiplex immunohistochemistry (mIF) is the best approach to predicting response. Figure reproduced from ref. 3, based on figure 2B, ref. 2.
The researchers concluded that, “The relative success of mIHC/IF in predicting patient response also provides insight into the spatial importance of tumor-immune interactions and the contribution of protein marker co-expression”. What was striking was that this success was achieved with an average of only 2–3 biomarkers, which suggests that exploiting the large multiplexing capacity of mIHC/mIF has the potential of improving diagnostic accuracy even more.
Staying ahead with spatial phenotyping
Spatial phenotyping certainly highlights the wealth of valuable data that can be extracted from a tissue slide. The power of this approach is so great that it has been suggested by Matt Humphries, scientific lead for Tissue Hybridization and Digital Pathology at Queen’s University Belfast, Northern Ireland, that labs that fail to include spatial phenotyping into their research run the risk of being left behind4.
Spatial phenotyping using multiplex IHC
Several multiplex imaging techniques have been developed to generate spatial multi-biomarker data from biopsies5. One approach is the automated multiplex system PhenoCycler-Fusion (formerly CODEX®; Akoya Technologies; https://www.leinco.com/codex_technology/) that uses DNA barcode technology comprised of unique oligonucleotide sequences conjugated to an antibody. An initial step involving staining tissue with up to 100 uniquely barcoded antibodies is followed by cycles of visualizing three antibodies at a time with reporter dyes linked to complimentary barcodes. This enables the visualization and quantification of dozens of biomarkers in a single tissue sample while maintaining cellular and sub-cellular detail.
Benefits of the system include:
- A comprehensive end-to-end solution containing the fluidics platform, assay reagents, and bioinformatics
- Benchtop footprint that integrates with an existing fluorescence microscope
- The system is scalable and capable of imaging up to 100 biomarkers per run
- Preserves the sample for region-of-interest (ROI) analysis and hematoxylin and eosin (H&E) staining
- Fresh frozen (FF) and formalin-fixed, paraffin-embedded (FFPE) tissue compatible
The system provides an accurate picture of cell neighborhoods and interactions in the tissue microenvironment and has been used in many studies involving spatial phenotyping in cancer research (for example, refs. 1 & 6).
Reliable and reproducible spatial phenotyping depends on high-quality antibodies
Confidence in data is critical and the reproducibility of analytical methods has become a major issue7, 8. The reproducibility of multiplex immunofluorescence (mIF) for spatial phenotyping depends on many factors, including antibody selection, antibody optimization and validation, panel design, staining optimization and validation, analysis strategies, and correct data generation9. One of the most important steps in ensuring reproducibility is the evaluation of the performance of antibodies in IHC. The International Working Group for Antibody Validation10, for example, proposed five approaches for antibody validation in general:
- genetic
- orthogonal
- independent antibody strategies
- expression of tagged proteins
- immunocapture followed by mass spectrometry
At least one of the five approaches should be used to validate an antibody for a particular application. The antibodies used in mIF should therefore be validated for the application and purified to ensure a high signal/noise ratio to ensure the fidelity of the analysis.
Click the button below to view our DNA barcoded antibodies and custom services for the PhenoCycler™-Fusion (CODEX)® Fluidics Instrument!
Conclusions
Spatial phenotyping is already delivering new insights into how cancer develops and progresses, providing ways to increase diagnostic accuracy, and guiding the development of innovative therapies such as immunotherapy. Confidence in results builds on many factors, including high quality antibodies that enable the generation of reliable and reproducible data.
Find out more about how spatial phenotyping is revolutionizing translational medicine and how Leinco Technologies is supporting these efforts with high quality antibodies:
References
- Phillips, D et al. Highly Multiplexed Phenotyping of Immunoregulatory Proteins in the Tumor Microenvironment by CODEX Tissue Imaging. Front. Immunol., 19 May 2021 https://www.ncbi.nlm.nih.gov/pmc/articles/PMC8170307/
- Lu S, et al. Comparison of Biomarker Modalities for Predicting Response to PD-1/PD-L1 Checkpoint Blockade: A Systematic Review and Meta-analysis. JAMA Oncol. 2019 Aug 1;5(8):1195-1204. doi: 10.1001/jamaoncol.2019.1549. PMID: 31318407; PMCID: PMC6646995.
- Blog post: Five publications that left their mark on spatial biology. Akoya Biosciences. https://www.akoyabio.com/blog/
- Staying Ahead of the Rest with Spatial Phenotyping. The Pathologist. 04/07/2021 https://thepathologist.com/diagnostics/staying-ahead-of-the-rest-with-spatial-phenotyping
- Allam, M. et al. Multiplex bioimaging of single-cell spatial profiles for precision cancer diagnostics and therapeutics. Precision Oncology volume 4, Article number: 11 (2020) https://www.nature.com/articles/s41698-020-0114-1
- Schürch, CM et al Coordinated Cellular Neighborhoods Orchestrate Antitumoral Immunity at the Colorectal Cancer Invasive Front Cell 182 (5), 3 September 2020, Pages 1341-1359.e19 https://www.sciencedirect.com/science/article/pii/S0092867420308709
- Baker M. 1,500 scientists lift the lid on reproducibility. Nature. 2016 May 26;533(7604):452-4. doi: 10.1038/533452a. https://www.nature.com/news/1-500-scientists-lift-the-lid-on-reproducibility-1.19970
- Freedman LP, et al. The Economics of Reproducibility in Preclinical Research. PLoS Biol. 2015 Jun 9;13(6):e1002165. doi: 10.1371/journal.pbio.1002165. eCollection 2015 Jun. http://journals.plos.org/plosbiology/article?id=10.1371/journal.pbio.1002165#sec010
- Laberiano-Fernández, C. et al. Best practices for technical reproducibility assessment of multiplex immunofluorescence. Front Mol Biosci. 2021 Aug 31;8:660202. doi: 10.3389/fmolb.2021.660202. eCollection 2021. PMID: 34532339
- Uhlén M, et al. A proposal for validation of antibodies. Nat Methods. 2016 Oct;13(10):823-7. doi: 10.1038/nmeth.3995. Epub 2016 Sep 5. PMID: 27595404.